基于搜索空间分割的协同进化遗传算法
郭方炜+许峰
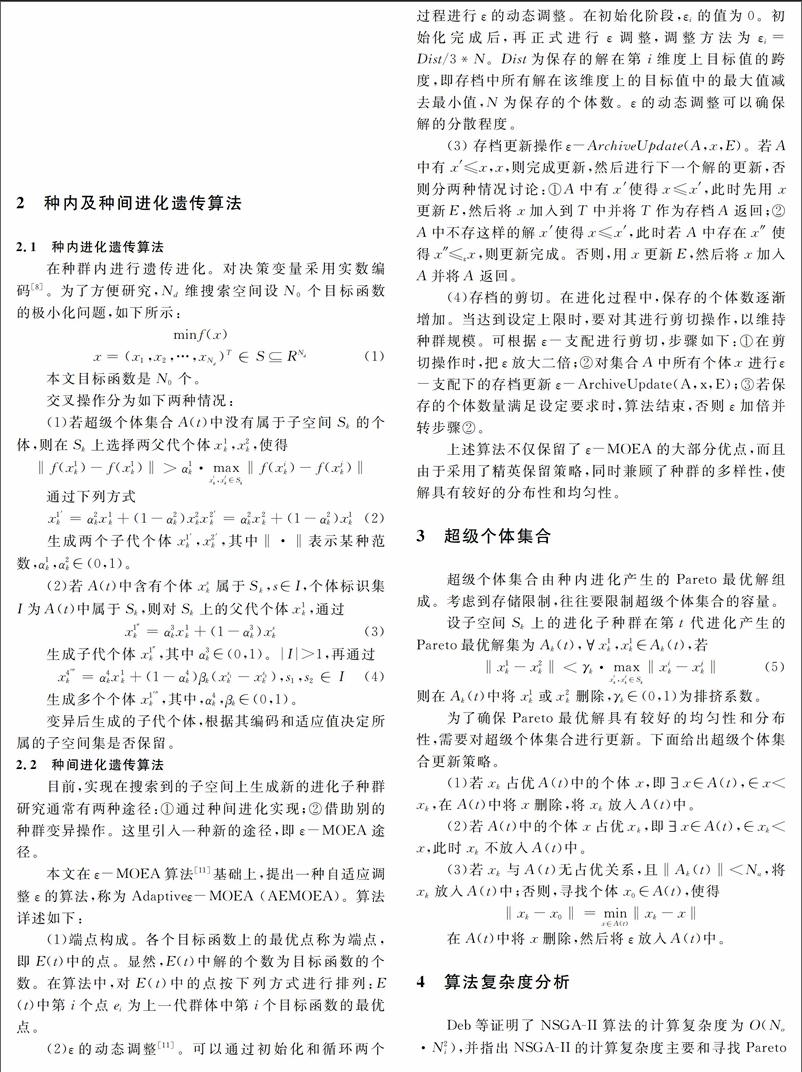
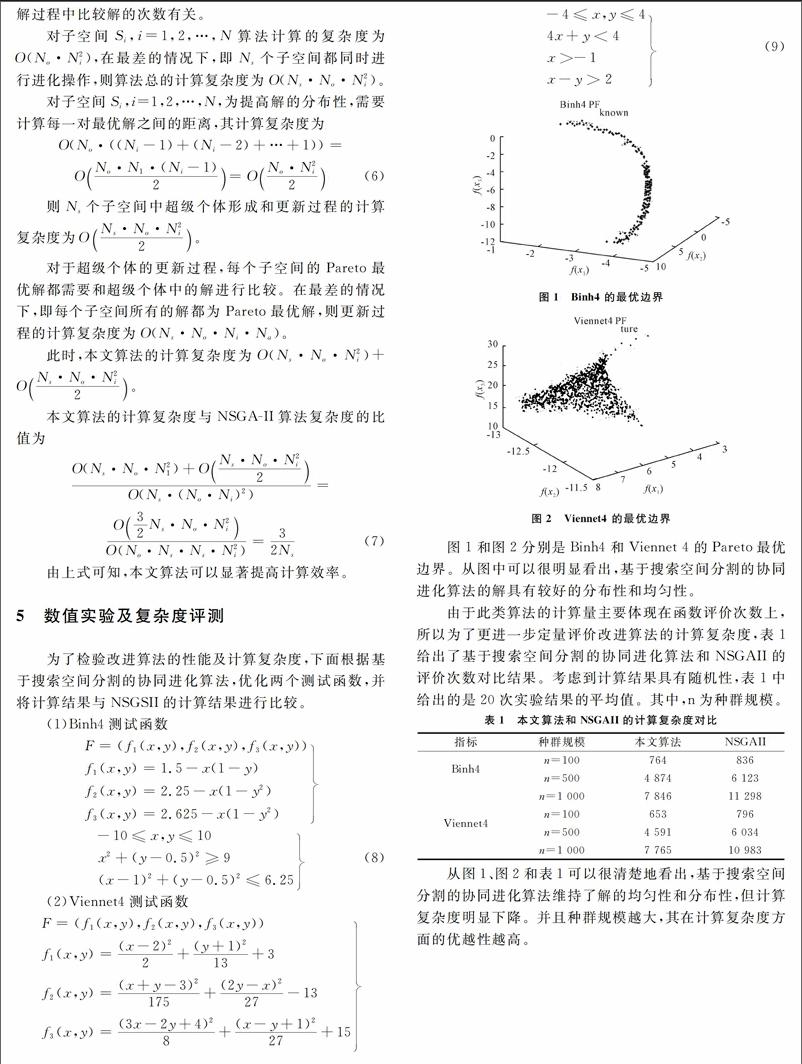
摘要:
针对经典协同进化遗传算法在优化大决策空间问题时计算复杂度较高的问题,提出了一种基于搜索空间分割的协同进化遗传算法,其基本思想是:将种群分割为不同规模的子种群,在进化过程中应用ε自适应方法调整子种群规模。复杂度分析和数值实验表明,改进后的算法可降低算法计算量,提高算法的优化效率。
關键词:
遗传算法;协同进化;空间分割;ε自适应调整;算法效率
DOIDOI:10.11907/rjdk.172249
中图分类号:TP312
文献标识码:A文章编号文章编号:16727800(2018)001009203
Abstract:In order to solve the problem of high computational complexity when the classical coevolutionary genetic algorithm is used to optimize the large decision space problem, a cooperative evolutionary genetic algorithm based on the search space segmentation is proposed. The basic idea is that the population is divided into sub populations with different scales, and the ε adaptive method is used to adjust the size of the sub population in the evolutionary process. Complexity analysis and numerical experiments show that the improved algorithm can reduce the computational complexity and optimize the efficiency of the algorithm.
Key Words:genetic algorithm; coevolution; spatial segmentation; adaptive adjustment; algorithm efficiency
0引言
基本遗传算法(Simple Genetic Algorithm,SGA)是20世纪70年代提出的一种基于自然进化的全局概率搜索优化算法[1],广泛应用于函数优化、组合优化、自动控制、图像处理、机器学习、数据挖掘等领域[23]。SGA的全局收敛性较好,但局部搜索能力相对不足,且在搜索过程中易陷于早熟收敛[4]。协同进化思想1964年被首次提出[5],20世纪90年代协同进化算法(coevolutionary algorithms,CEA)被提出[67]。目前,协同进化算法已成功应用到作业调度、人工神经网络、模式识别和工程设计优化等领域[710]。
经典协同进化遗传算法在进行空间分割时,没有采用合适的方法控制种内及种间进化,无法控制子种群的规模,导致算法复杂度较高。本文在已有工作的基础上,提出了基于搜索空间分割的改进协同进化遗传算法,从理论上分析了算法的复杂度,并用数值实验测评了算法性能。
1空间分割
遗传算法是一种基于生物遗传和进化优化的算法,其基本思想是:交叉机制能实现子种群优化问题的全局搜索,优化问题的局部搜索能利用变异机制实现,但是交叉和变异机制很难通过传统算法协调,易陷于局部最优解。针对这一现象,提出一种改进的多种群遗传算法。
本文根据多目标优化问题的特点,提出了基于搜索空间分割的多种群协同进化算法,阐述了算法思想、搜索空间分割方法、遗传算法设计、超级个体集合的形成和更新策略以及种群的生成途径等,并分析了算法计算的复杂性。
空间分割算法思想是:首先将较大的搜索空间分割为多个子空间,然后在每个子空间上由一个子种群不断进化优化,分别将不同的遗传算法应用到子种群内部和子种群之间,由遗传算法运算产生新的种群和新的个体,以其覆盖成新的子空间;采用Pareto最优解[7]提交方法、超级个体集合更新策略,以保证高效找到Pareto最优解。
对于多目标优化问题中的整个搜索空间而言,子空间应该是完备的,每个子空间之间最好是隔离的,整个搜索空间S的分割可用S1,S2,…,SNS表示,其中,子空间的数目设定为NS,则有∪Nsi=1Si=S,Si∩Sj=,1≤i,j≤Ns。
6结语
本文从空间分割的角度出发,提出了空间规模自适应调整的协同进化遗传算法。复杂度的理论分析和数值实验结果均表明:与经典协同进化遗传算法相比,改进后的算法在一定程度上降低了解的计算复杂度。
由于协同进化算法的理论体系尚不成熟,算法性能很难从理论层面进行证明,而只能根据对比实验加以说明。提高经典协同进化遗传算法优化效率的方法有多种,如组织进化、引进多智能体等。本文采用的空间分割方法与其它方法相比,优点是可以与自适应调整方法相结合,缺点是对解的质量基本没有改进,这完全符合优化中的“没有免费的午餐定理(No Free Lunch, NFL)”。
参考文献:
[1]HOLLAND J H.Adaptation in natural and artificial systems [M].Cambridge(USA):MIT Press,1975(41):559577.
[2]GOLDBERG D E.Genetic algorithms in search,optimization.and machine learning[M].New Jersey:Addison Wesley Publishing Company,1989.
[3]PLANT W R,SCHAEFER G,NAKASHIMA T.An overview of genetic algorithms in simulation soccer[C].2008 IEEE Congress on Evolutionary Computation.Hong Kong:IEEE Press,2008:38983905.
[4]CAO XIAN BIN,LUO WENJIAN,WANG XIFA.A coevo1ution pattern based on ecological population competition model[J].Journal of Software,2001,12(4):556562.
[5]EHDICH P R,RAVEN P H.Butterflies and plants:a study in evolution[J].Evolution,1964(18):586608..
[6]ROSIN C D,BELEW R K.New methods for competitive evolution[J].Evolutionary Computation,1997,5(1):129.
[7]CARTLIDGE J,BULLOCK S.Combating evolutionary disengagement by reducing parasite virulence[J].Evolutionary Computation,2002,12(2):193222.
[8]JONG K A DE.The analysis of the behavior of a class of genetic adaptive system[D].Michigan:University of Michigan,1975.
[9]SCHAFFER J D,CARUANA R A,ESHELMAN L J.A study of control parameters affecting online performance of genetic algorithms for function optimization[C].Proceedings of the 3rd International Conference on Genetic Algorithms.Los Altos(USA):Morgan Kaufmann Publish,1989:5160.
[10]DONG HONGBIN,HUANG HOUKUAN,YIN GUISHENG,et al.An overview of the research on evolutionary algorithms[J].Journal of Computer Research and Development,2008,45(3):454463.
[11]AGUIRRE H, TANAKA K.Adaptive εranking on manyobjective problems [J].Evolutionary Intelligence, 2009(2):183206.
(責任编辑:杜能钢)
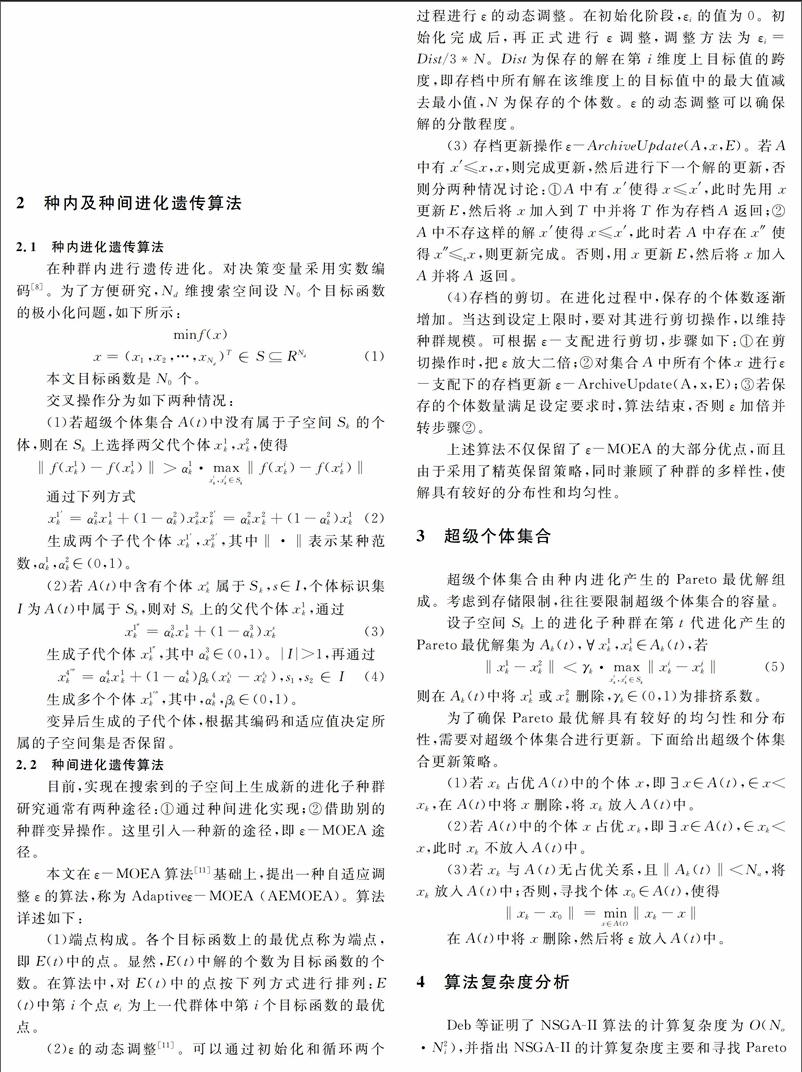
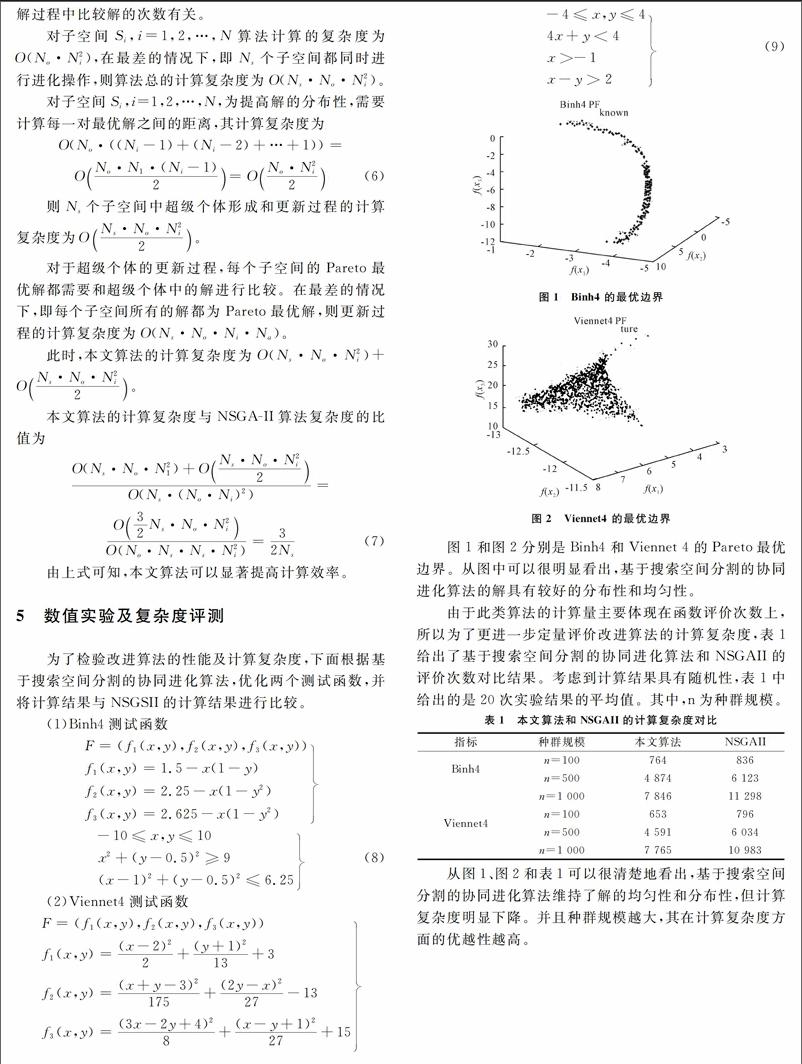
摘要:
针对经典协同进化遗传算法在优化大决策空间问题时计算复杂度较高的问题,提出了一种基于搜索空间分割的协同进化遗传算法,其基本思想是:将种群分割为不同规模的子种群,在进化过程中应用ε自适应方法调整子种群规模。复杂度分析和数值实验表明,改进后的算法可降低算法计算量,提高算法的优化效率。
關键词:
遗传算法;协同进化;空间分割;ε自适应调整;算法效率
DOIDOI:10.11907/rjdk.172249
中图分类号:TP312
文献标识码:A文章编号文章编号:16727800(2018)001009203
Abstract:In order to solve the problem of high computational complexity when the classical coevolutionary genetic algorithm is used to optimize the large decision space problem, a cooperative evolutionary genetic algorithm based on the search space segmentation is proposed. The basic idea is that the population is divided into sub populations with different scales, and the ε adaptive method is used to adjust the size of the sub population in the evolutionary process. Complexity analysis and numerical experiments show that the improved algorithm can reduce the computational complexity and optimize the efficiency of the algorithm.
Key Words:genetic algorithm; coevolution; spatial segmentation; adaptive adjustment; algorithm efficiency
0引言
基本遗传算法(Simple Genetic Algorithm,SGA)是20世纪70年代提出的一种基于自然进化的全局概率搜索优化算法[1],广泛应用于函数优化、组合优化、自动控制、图像处理、机器学习、数据挖掘等领域[23]。SGA的全局收敛性较好,但局部搜索能力相对不足,且在搜索过程中易陷于早熟收敛[4]。协同进化思想1964年被首次提出[5],20世纪90年代协同进化算法(coevolutionary algorithms,CEA)被提出[67]。目前,协同进化算法已成功应用到作业调度、人工神经网络、模式识别和工程设计优化等领域[710]。
经典协同进化遗传算法在进行空间分割时,没有采用合适的方法控制种内及种间进化,无法控制子种群的规模,导致算法复杂度较高。本文在已有工作的基础上,提出了基于搜索空间分割的改进协同进化遗传算法,从理论上分析了算法的复杂度,并用数值实验测评了算法性能。
1空间分割
遗传算法是一种基于生物遗传和进化优化的算法,其基本思想是:交叉机制能实现子种群优化问题的全局搜索,优化问题的局部搜索能利用变异机制实现,但是交叉和变异机制很难通过传统算法协调,易陷于局部最优解。针对这一现象,提出一种改进的多种群遗传算法。
本文根据多目标优化问题的特点,提出了基于搜索空间分割的多种群协同进化算法,阐述了算法思想、搜索空间分割方法、遗传算法设计、超级个体集合的形成和更新策略以及种群的生成途径等,并分析了算法计算的复杂性。
空间分割算法思想是:首先将较大的搜索空间分割为多个子空间,然后在每个子空间上由一个子种群不断进化优化,分别将不同的遗传算法应用到子种群内部和子种群之间,由遗传算法运算产生新的种群和新的个体,以其覆盖成新的子空间;采用Pareto最优解[7]提交方法、超级个体集合更新策略,以保证高效找到Pareto最优解。
对于多目标优化问题中的整个搜索空间而言,子空间应该是完备的,每个子空间之间最好是隔离的,整个搜索空间S的分割可用S1,S2,…,SNS表示,其中,子空间的数目设定为NS,则有∪Nsi=1Si=S,Si∩Sj=,1≤i,j≤Ns。
6结语
本文从空间分割的角度出发,提出了空间规模自适应调整的协同进化遗传算法。复杂度的理论分析和数值实验结果均表明:与经典协同进化遗传算法相比,改进后的算法在一定程度上降低了解的计算复杂度。
由于协同进化算法的理论体系尚不成熟,算法性能很难从理论层面进行证明,而只能根据对比实验加以说明。提高经典协同进化遗传算法优化效率的方法有多种,如组织进化、引进多智能体等。本文采用的空间分割方法与其它方法相比,优点是可以与自适应调整方法相结合,缺点是对解的质量基本没有改进,这完全符合优化中的“没有免费的午餐定理(No Free Lunch, NFL)”。
参考文献:
[1]HOLLAND J H.Adaptation in natural and artificial systems [M].Cambridge(USA):MIT Press,1975(41):559577.
[2]GOLDBERG D E.Genetic algorithms in search,optimization.and machine learning[M].New Jersey:Addison Wesley Publishing Company,1989.
[3]PLANT W R,SCHAEFER G,NAKASHIMA T.An overview of genetic algorithms in simulation soccer[C].2008 IEEE Congress on Evolutionary Computation.Hong Kong:IEEE Press,2008:38983905.
[4]CAO XIAN BIN,LUO WENJIAN,WANG XIFA.A coevo1ution pattern based on ecological population competition model[J].Journal of Software,2001,12(4):556562.
[5]EHDICH P R,RAVEN P H.Butterflies and plants:a study in evolution[J].Evolution,1964(18):586608..
[6]ROSIN C D,BELEW R K.New methods for competitive evolution[J].Evolutionary Computation,1997,5(1):129.
[7]CARTLIDGE J,BULLOCK S.Combating evolutionary disengagement by reducing parasite virulence[J].Evolutionary Computation,2002,12(2):193222.
[8]JONG K A DE.The analysis of the behavior of a class of genetic adaptive system[D].Michigan:University of Michigan,1975.
[9]SCHAFFER J D,CARUANA R A,ESHELMAN L J.A study of control parameters affecting online performance of genetic algorithms for function optimization[C].Proceedings of the 3rd International Conference on Genetic Algorithms.Los Altos(USA):Morgan Kaufmann Publish,1989:5160.
[10]DONG HONGBIN,HUANG HOUKUAN,YIN GUISHENG,et al.An overview of the research on evolutionary algorithms[J].Journal of Computer Research and Development,2008,45(3):454463.
[11]AGUIRRE H, TANAKA K.Adaptive εranking on manyobjective problems [J].Evolutionary Intelligence, 2009(2):183206.
(責任编辑:杜能钢)